5. 映画レビューデータを通したデータ処理演習¶
参考サイト: Collaborative Filtering for Movie Recommendations
5.1. ゴール¶
CSV/TSV等のテキストファイルで用意された生のデータの読み込み方、そこから必要な項目を抽出するようなデータ処理、平均値・分散値を算出したりといった一般的な統計処理、グラフや分布図等の描画方法について学んでみましょう。
なお、MovieLensと呼ばれる映画レビューデータセットを元に、似ているユーザを探し出し、そのユーザが高評価を付けているまだ視聴していない映画を抽出する映画推薦の例も示します。重要なのは個々のコードを覚えることではなく、必要なことを自身で検索して探し出し、参考にして実装できるようになることです。
例えば pandas cheat sheet
ぐらいでググるとPandas Cheat Sheet for Data Science in Pythonが見つかります。pandas 列抽出ならこういう結果が得られます。検索上手になろう。
5.2. 利用パッケージと公式ドキュメントやチュートリアル¶
すべてを使いこなすのではなく、必要な時に必要なものを探し出せるようになろう。
Python標準モジュール
外部パッケージ
requests: HTTPライブラリ。
numpy: 数値演算・行列演算ライブラリ。
pandas: 表形式データ操作ライブラリ。
matplotlib: 可視化ライブラリ。
seaborn: 可視化ライブラリ。
Linuxコマンド
ls: ファイル一覧をリスト表示。Windowsのdir相当。
tree: 階層構造を可視化。
5.3. データセットの準備¶
5.3.1. データセットのダウンロード¶
今回利用するデータセットは MovieLens の最小データセットを利用する。MovieLensは、視聴者による映画に対する5点満点のレビューを収集して構築したものであり、「ユーザ1番は映画500番に対して4点を付けた」というデータの集合になっている。またユーザの嗜好や映画の属性についてもある程度判断するための情報として、ユーザは年齢・性別、映画はタイトル・カテゴリ・出版年が付与されている。
#!curl -O http://files.grouplens.org/datasets/movielens/ml-latest-small.zip
!apt install tree
#!rm -rf *
#import requests, zipfile, io
movielens_zipfile_url = "http://files.grouplens.org/datasets/movielens/ml-latest-small.zip"
save_path = "./"
r = requests.get(movielens_zipfile_url)
print(r)
print(type(r))
print(r.content)
zfile = zipfile.ZipFile(io.BytesIO(r.content))
zfile.extractall(save_path)
!ls
!ls ml-latest-small/*
!tree
Reading package lists... Done
Building dependency tree
Reading state information... Done
The following NEW packages will be installed:
tree
0 upgraded, 1 newly installed, 0 to remove and 30 not upgraded.
Need to get 40.7 kB of archives.
After this operation, 105 kB of additional disk space will be used.
Get:1 http://archive.ubuntu.com/ubuntu bionic/universe amd64 tree amd64 1.7.0-5 [40.7 kB]
Fetched 40.7 kB in 1s (69.2 kB/s)
Selecting previously unselected package tree.
(Reading database ... 160980 files and directories currently installed.)
Preparing to unpack .../tree_1.7.0-5_amd64.deb ...
Unpacking tree (1.7.0-5) ...
Setting up tree (1.7.0-5) ...
Processing triggers for man-db (2.8.3-2ubuntu0.1) ...
IOPub data rate exceeded.
The notebook server will temporarily stop sending output
to the client in order to avoid crashing it.
To change this limit, set the config variable
`--NotebookApp.iopub_data_rate_limit`.
Current values:
NotebookApp.iopub_data_rate_limit=1000000.0 (bytes/sec)
NotebookApp.rate_limit_window=3.0 (secs)
ml-latest-small/links.csv ml-latest-small/README.txt
ml-latest-small/movies.csv ml-latest-small/tags.csv
ml-latest-small/ratings.csv
.
├── ml-latest-small
│ ├── links.csv
│ ├── movies.csv
│ ├── ratings.csv
│ ├── README.txt
│ └── tags.csv
└── sample_data
├── anscombe.json
├── california_housing_test.csv
├── california_housing_train.csv
├── mnist_test.csv
├── mnist_train_small.csv
└── README.md
2 directories, 11 files
5.3.2. 外部データの利用方法¶
上記ではGoogle Colab実行時に自動で用意される環境内にデータをダウンロードしている。これ以外にもローカルPCのファイルをアップロードしたり、Googleドライブを利用する方法もある。ここでは参考サイトの紹介にとどめる。いろいろ試して好みの方法を利用しよう。
5.3.3. CSVファイルをpd.DataFrameに変換し覗いてみる¶
pandasではデータセットをDataFrame型と呼ばれる二次元表形式の構造でデータを管理し、処理することが多い。
表をExcelのような表計算ソフトで処理する場合には「行方向インデックス(1〜N)」「列方向インデックス(A〜AZ,,,)」を用いて対象セルを指定し、平均値や分散を求めたり、特定条件に合致する値を抽出して処理することになる。
ここでは代表的な行・列指定方法、値の参照方法を眺めていこう。
参考: ml-latest-small詳細: ml-latest-small-README.html
tips
pd.reac_csv('filename')
でCSVやTSV形式のファイルを読み込むことができる。なおファイル冒頭に列名が記載されている場合にはそれを列名として読み取ることもできるし、逆にそれをスキップすることもできる。詳細はドキュメントで確認しよう。DataFrameに対して
head()
で冒頭5個までのデータを眺めることができる。大規模データを全出力しても意味がないため、ざっくりと確認したい場合に便利。今回のデータセットは以下の4つのデータフレームで構成されている。これらを組み合わせて利用すると、例えば「movieId==1は’Toy Story (1995)’で、そのカテゴリは Adventure等複数が付与されている。より詳細情報はimdbで114709から参照できる。この映画に対してuserId==1は4点を付けている」といったことを読み出すことができる。たかだか4つのファイルでも頭の中で組み合わせて考えるのは難しいので、自分なりに図を書いてどう関連しているかを鳥瞰しやすくしよう。
links: movieId, imdbId, tmdbId
movies: movieId, title, genres
ratings: userId, movieId, rating, timestamp
tags: userId, movieId, tag, timestamp
movieId | title | genres | |
---|---|---|---|
0 | 1 | Toy Story (1995) | Adventure|Animation|Children|Comedy|Fantasy |
1 | 2 | Jumanji (1995) | Adventure|Children|Fantasy |
2 | 3 | Grumpier Old Men (1995) | Comedy|Romance |
3 | 4 | Waiting to Exhale (1995) | Comedy|Drama|Romance |
4 | 5 | Father of the Bride Part II (1995) | Comedy |
userId | movieId | rating | timestamp | |
---|---|---|---|---|
0 | 1 | 1 | 4.0 | 964982703 |
1 | 1 | 3 | 4.0 | 964981247 |
2 | 1 | 6 | 4.0 | 964982224 |
3 | 1 | 47 | 5.0 | 964983815 |
4 | 1 | 50 | 5.0 | 964982931 |
5.4. 行や列を指定した簡易処理¶
5.4.1. 特定の行(サンプル)や列(特徴)を指定して眺めてみる¶
moviesはmovieId, title, genresの3要素で構成されるデータが並んでいる。ここではtitleだけを指定し、かつ、冒頭5番目までのデータを出力指定している。
tips
DataFrameに対して
['列名']
で列を指定できる。DataFrameに対して
[start:end]
のようにスライス処理(これはPythonのリストに対する処理と同等)を記述することで、連続した複数行を指定できる。startを省略した場合は冒頭から、endを省略した場合は最後までという意味になる。
movieId title \
0 1 Toy Story (1995)
1 2 Jumanji (1995)
2 3 Grumpier Old Men (1995)
3 4 Waiting to Exhale (1995)
4 5 Father of the Bride Part II (1995)
5 6 Heat (1995)
genres
0 Adventure|Animation|Children|Comedy|Fantasy
1 Adventure|Children|Fantasy
2 Comedy|Romance
3 Comedy|Drama|Romance
4 Comedy
5 Action|Crime|Thriller
5.4.2. 条件を指定して眺めてみる1(userId == 1 のレーティングを確認)¶
userId movieId rating timestamp
0 1 1 4.0 964982703
1 1 3 4.0 964981247
2 1 6 4.0 964982224
3 1 47 5.0 964983815
4 1 50 5.0 964982931
.. ... ... ... ...
227 1 3744 4.0 964980694
228 1 3793 5.0 964981855
229 1 3809 4.0 964981220
230 1 4006 4.0 964982903
231 1 5060 5.0 964984002
[232 rows x 4 columns]
5.4.3. 数値情報の概要(出現回数・平均・分散・最小値・最大値・四分位数)を眺めてみる¶
userId | movieId | rating | timestamp | |
---|---|---|---|---|
count | 100836.000000 | 100836.000000 | 100836.000000 | 1.008360e+05 |
mean | 326.127564 | 19435.295718 | 3.501557 | 1.205946e+09 |
std | 182.618491 | 35530.987199 | 1.042529 | 2.162610e+08 |
min | 1.000000 | 1.000000 | 0.500000 | 8.281246e+08 |
25% | 177.000000 | 1199.000000 | 3.000000 | 1.019124e+09 |
50% | 325.000000 | 2991.000000 | 3.500000 | 1.186087e+09 |
75% | 477.000000 | 8122.000000 | 4.000000 | 1.435994e+09 |
max | 610.000000 | 193609.000000 | 5.000000 | 1.537799e+09 |
userId | movieId | rating | timestamp | |
---|---|---|---|---|
count | 232.0 | 232.000000 | 232.000000 | 2.320000e+02 |
mean | 1.0 | 1854.603448 | 4.366379 | 9.649856e+08 |
std | 0.0 | 1055.962726 | 0.800048 | 4.841309e+04 |
min | 1.0 | 1.000000 | 1.000000 | 9.649805e+08 |
25% | 1.0 | 1047.250000 | 4.000000 | 9.649817e+08 |
50% | 1.0 | 1960.500000 | 5.000000 | 9.649824e+08 |
75% | 1.0 | 2641.750000 | 5.000000 | 9.649830e+08 |
max | 1.0 | 5060.000000 | 5.000000 | 9.657197e+08 |
userId | movieId | rating | timestamp | |
---|---|---|---|---|
count | 232.0 | 232.00 | 232.00 | 2.32e+02 |
mean | 1.0 | 1854.60 | 4.37 | 9.65e+08 |
std | 0.0 | 1055.96 | 0.80 | 4.84e+04 |
min | 1.0 | 1.00 | 1.00 | 9.65e+08 |
25% | 1.0 | 1047.25 | 4.00 | 9.65e+08 |
50% | 1.0 | 1960.50 | 5.00 | 9.65e+08 |
75% | 1.0 | 2641.75 | 5.00 | 9.65e+08 |
max | 1.0 | 5060.00 | 5.00 | 9.66e+08 |
5.5. 大雑把に可視化してみる¶
tips
5.5.1. pd.plotでグラフ描画¶
5.5.2. 条件絞り込んでpd.plot¶
userId movieId rating timestamp
0 1 1 4.0 964982703
516 5 1 4.0 847434962
874 7 1 4.5 1106635946
1434 15 1 2.5 1510577970
1667 17 1 4.5 1305696483
... ... ... ... ...
97364 606 1 2.5 1349082950
98479 607 1 4.0 964744033
98666 608 1 2.5 1117408267
99497 609 1 3.0 847221025
99534 610 1 5.0 1479542900
[215 rows x 4 columns]
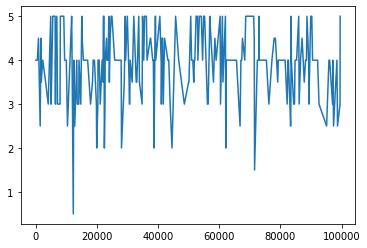
5.5.3. pd.plot(kind=’hist’)でヒストグラム¶
5.5.4. seaborn.boxplot で箱ひげ図¶
5.6. テーブルを組み合わせるてみる¶
ユーザを行、列を映画とする行列を作成し、各要素の値をratingとする(ない場合にはNoneにする)。これをユーザ・映画行列と呼ぶことにしよう。ユーザ・映画行列を用意できれば「同じ映画集合に対して高評価を付けているユーザグループ」を抽出できるかもしれない。そのようなグループを抽出できたならば「同じグループが高評価しているが、まだ評価されていない映画」を発見することで高評価を得やすい映画を推薦できるだろう。このように映画の視聴履歴を元に推薦する方法を協調フィルタリングと呼ぶ。
今は詳細を省くが、以下の手順で視聴履歴を利用してみよう。
step 1: ユーザ・映画行列を作成。
step 2: ユーザ間の類似度を算出。
step 3: あるユーザiに対する最大類似度となるユーザjを抽出。
step 4: ユーザjが高く評価している映画をリストアップ。
step 5: リストアップした映画からユーザiが見ていないもの(=推薦候補)を抽出。
5.6.1. step 1: ユーザ・映画行列を作成。¶
NaN
は Not a Number の略。数値ではない値のこと。ここでは該当要素に値がなかった場合のデフォルト値として設定されている。pd.pivot_tableでピボットテーブルを作成。
movieId | 1 | 2 | 3 | 4 | 5 | ... | 193581 | 193583 | 193585 | 193587 | 193609 |
---|---|---|---|---|---|---|---|---|---|---|---|
userId | |||||||||||
1 | 4.0 | NaN | 4.0 | NaN | NaN | ... | NaN | NaN | NaN | NaN | NaN |
2 | NaN | NaN | NaN | NaN | NaN | ... | NaN | NaN | NaN | NaN | NaN |
3 | NaN | NaN | NaN | NaN | NaN | ... | NaN | NaN | NaN | NaN | NaN |
4 | NaN | NaN | NaN | NaN | NaN | ... | NaN | NaN | NaN | NaN | NaN |
5 | 4.0 | NaN | NaN | NaN | NaN | ... | NaN | NaN | NaN | NaN | NaN |
... | ... | ... | ... | ... | ... | ... | ... | ... | ... | ... | ... |
606 | 2.5 | NaN | NaN | NaN | NaN | ... | NaN | NaN | NaN | NaN | NaN |
607 | 4.0 | NaN | NaN | NaN | NaN | ... | NaN | NaN | NaN | NaN | NaN |
608 | 2.5 | 2.0 | 2.0 | NaN | NaN | ... | NaN | NaN | NaN | NaN | NaN |
609 | 3.0 | NaN | NaN | NaN | NaN | ... | NaN | NaN | NaN | NaN | NaN |
610 | 5.0 | NaN | NaN | NaN | NaN | ... | NaN | NaN | NaN | NaN | NaN |
610 rows × 9724 columns
movieId | 1 | 2 | 3 | 4 | 5 | ... | 193581 | 193583 | 193585 | 193587 | 193609 |
---|---|---|---|---|---|---|---|---|---|---|---|
count | 215.00 | 110.00 | 52.00 | 7.00 | 49.00 | ... | 1.0 | 1.0 | 1.0 | 1.0 | 1.0 |
mean | 3.92 | 3.43 | 3.26 | 2.36 | 3.07 | ... | 4.0 | 3.5 | 3.5 | 3.5 | 4.0 |
std | 0.83 | 0.88 | 1.05 | 0.85 | 0.91 | ... | NaN | NaN | NaN | NaN | NaN |
min | 0.50 | 0.50 | 0.50 | 1.00 | 0.50 | ... | 4.0 | 3.5 | 3.5 | 3.5 | 4.0 |
25% | 3.50 | 3.00 | 3.00 | 1.75 | 3.00 | ... | 4.0 | 3.5 | 3.5 | 3.5 | 4.0 |
50% | 4.00 | 3.50 | 3.00 | 3.00 | 3.00 | ... | 4.0 | 3.5 | 3.5 | 3.5 | 4.0 |
75% | 4.50 | 4.00 | 4.00 | 3.00 | 3.50 | ... | 4.0 | 3.5 | 3.5 | 3.5 | 4.0 |
max | 5.00 | 5.00 | 5.00 | 3.00 | 5.00 | ... | 4.0 | 3.5 | 3.5 | 3.5 | 4.0 |
8 rows × 9724 columns
5.6.2. step 2: ユーザ間の類似度を算出。¶
ユーザ間類似度は「評価値の二乗誤差」を用いることにしよう。同じ映画を見ているとは限らないため、視聴映画リストで重複している映画だけを抽出し、誤差を算出する必要がある。
df.notnullで欠損値を除外。
df.indexで該当列インデックスを参照。
set.intersectionで共通集合を抽出。
np.arrayでnumpy用配列(ndarray)型に変換。
np.dotで内積計算。
np.linalg.normでベクトルのノルム(大きさ)計算。
movieId
1 4.0
2 NaN
3 4.0
4 NaN
5 NaN
...
193581 NaN
193583 NaN
193585 NaN
193587 NaN
193609 NaN
Name: 1, Length: 9724, dtype: float64
movieId
1 True
2 False
3 True
4 False
5 False
...
193581 False
193583 False
193585 False
193587 False
193609 False
Name: 1, Length: 9724, dtype: bool
movieId
1 4.0
3 4.0
6 4.0
47 5.0
50 5.0
...
3744 4.0
3793 5.0
3809 4.0
4006 4.0
5060 5.0
Name: 1, Length: 232, dtype: float64
Int64Index([ 1, 3, 6, 47, 50, 70, 101, 110, 151, 157,
...
3671, 3702, 3703, 3729, 3740, 3744, 3793, 3809, 4006, 5060],
dtype='int64', name='movieId', length=232)
# ユーザ間類似度は「評価値の二乗誤差」を用いることにしよう。
# 同じ映画を見ているとは限らないため、
# 視聴映画リストで重複している映画だけを抽出し、誤差を算出する必要がある。
def get_similarity(person1, person2, dataset):
# person1とperson2の映画リスト
movies1 = dataset.loc[person1][dataset.loc[person1].notnull()].index
movies2 = dataset.loc[person2][dataset.loc[person2].notnull()].index
# 共通映画リスト
movies1 = set(movies1)
movies2 = set(movies2)
both_movies = movies1.intersection(movies2)
# 共通リストがない場合
if len(both_movies) == 0:
return 0
#print('{}, {}: len(person1)={}, len(person2)={}, len(both_movies)={}'.format(person1, person2, len(movies1), len(movies2), len(both_movies)))
# 共通リストがある場合
vector1 = np.array(dataset.loc[person1][both_movies])
vector2 = np.array(dataset.loc[person2][both_movies])
#print(type(vector1), vector1.shape)
nominator = np.dot(vector1, vector2)
denominator = np.linalg.norm(vector1) * np.linalg.norm(vector2)
similarity = nominator / denominator
return similarity
print(get_similarity(1, 1, df)) #同じユーザ同士なら類似度1
print(get_similarity(1, 2, df))
print(get_similarity(2, 1, df)) #違うユーザを順番入れ替えて測っても同じ類似度が出ることを確認
5.6.3. step 3: あるユーザiに対する最大類似度となるユーザjを抽出。¶
def get_similarities_top_k(source, dataset, k=3):
targets = list(dataset.index) # ユーザインデックスを取得
# 類似度保存用配列を用意
# 配列長を+1しているのは1番目から使うため。
similarities = np.zeros(len(dataset)+1)
# 全ユーザとの類似度算出
for opponent in targets:
if opponent == source:
similarities[opponent] = 0. #自分自身は無視する
else:
similarities[opponent] = get_similarity(source, opponent, dataset)
# 降順ソートして上位k人のidを取得
indices, values = get_top_k(similarities, k)
return indices, values
# userId==1との類似度上位ユーザを確認
k = 3
indices, values = get_similarities_top_k(1, df, k)
print('k', k)
print(indices)
print(values)
5.6.4. step 4: ユーザjが高く評価している映画をリストアップ。¶
movieId
150 4.0
539 4.0
903 4.0
1196 3.0
1234 3.0
Name: 383, dtype: float64
Int64Index([ 150, 539, 903, 1196, 1234, 1246, 1293, 1299, 1584, 1953, 1994,
2324, 2396, 2436, 2445, 2447, 2496, 2501, 2506, 2539, 2541, 2546,
2558, 2686, 2690, 2712, 2762, 2881, 2906, 2961, 2977, 2996, 3006,
3115],
dtype='int64', name='movieId')
def get_top_movies(target, df):
# 対象ユーザの評価とそのインデックス
user_ratings = df.loc[target][df.loc[target].notnull()]
user_ratings_indices = df.loc[target][df.loc[target].notnull()].index
# 対象ユーザの上位評価
relative_indices, values = get_top_k(np.array(user_ratings))
true_indices = user_ratings_indices[relative_indices]
return true_indices, user_ratings[true_indices]
opponent = 383
indices, values = get_top_movies(opponent, df)
print(indices)
print(values)
5.6.5. step 5: リストアップした映画からユーザiが見ていないもの(=推薦候補)を抽出。¶
{1024, 1, 1025, 3, 2048, 1029, 6, 1030, 1031, 1032, 2054, 2058, 2571, 527, 1552, 1042, 2580, 1049, 2078, 543, 3617, 1060, 1573, 2596, 552, 553, 2090, 1580, 2093, 2094, 47, 2096, 1073, 50, 1587, 2099, 3639, 1080, 2105, 2616, 2617, 1089, 1090, 2115, 1092, 2116, 70, 2628, 1097, 3147, 590, 592, 593, 1617, 2640, 596, 1620, 2641, 2644, 2648, 1625, 2137, 2139, 3671, 2141, 2654, 2143, 608, 2657, 3168, 101, 1127, 3176, 1644, 110, 1136, 2161, 3702, 3703, 2174, 2692, 648, 1676, 2700, 2193, 3729, 661, 151, 2716, 157, 3740, 3744, 673, 163, 3243, 1196, 1197, 1198, 3247, 3253, 1206, 1208, 1210, 1213, 1214, 1219, 1220, 1732, 1222, 1224, 2761, 1226, 3273, 2253, 3793, 216, 1240, 2268, 733, 223, 736, 2273, 3809, 231, 1256, 1258, 235, 2797, 1265, 1777, 2291, 1270, 1275, 1278, 1793, 1282, 260, 2826, 1291, 780, 1804, 1805, 1298, 2329, 2338, 804, 296, 2858, 2353, 2872, 3386, 316, 2366, 1348, 333, 2387, 2899, 2389, 2395, 349, 1377, 356, 2916, 2406, 362, 2414, 367, 3439, 3440, 3441, 1396, 3448, 3450, 2427, 1408, 1920, 2944, 2947, 2948, 2949, 1927, 2959, 2450, 919, 3479, 923, 2459, 3489, 1954, 1445, 2470, 423, 4006, 2985, 2987, 940, 2478, 943, 1967, 2991, 2993, 2997, 441, 954, 2492, 1473, 5060, 2502, 3527, 457, 2000, 2005, 3033, 3034, 1500, 2012, 480, 2528, 2018, 2529, 2028, 1517, 2542, 3052, 3053, 1009, 2033, 500, 3062, 3578, 2046, 1023}
{2396, 2324, 1246}
{2396, 2324, 1246}
def get_recommendation(source, opponent_indices, df):
# userId==sourceの視聴映画id
source_indices = set(df.loc[source][df.loc[source].notnull()].index)
# 対象ユーザの高評価映画id
opponent_indices = set(opponent_indices)
# 高評価idのうち、userId==sourceがまだ視聴していないid
recommend_indices = opponent_indices - source_indices
return recommend_indices
userId = 1
opponent = 383
opponent_indices, values = get_top_movies(opponent, df)
recommend_indices = get_recommendation(userId, opponent_indices, df)
print(recommend_indices)
userId = 1
opponent = 388
opponent_indices, values = get_top_movies(opponent, df)
recommend_indices = get_recommendation(userId, opponent_indices, df)
print(recommend_indices)