SVDによる圧縮の例(not 次元削減)¶
全体の流れ
画像ファイルをグレイスケールに変換する。(そのままだとR, G, Bの3レイヤー分の行列があるので、今回はグレイスケールに変換して1つの行列として扱う)
グレイスケールが保存されている行列を特異値分解する。
分解によりどのぐらい近似できているのかを確認する。
近似度合いがどのぐらいあるのかを可視化して確認する。
画像データの準備¶
画像データのshape確認¶
グレイスケール変換¶
grayscale=0.2126R+0.7152G+0.0722B
特異値分解¶
sを対角行列に変換¶
sは対角行列。正方行列ならnp.diag()で変換できるが、特異値分解で出てくる特異値は行列サイズが異る(U, Vtに合わせる必要がある)。そこで、サイズを指定したゼロ行列を作成して対角成分のみコピーすることで作成している。
圧縮してみる¶
特異値の大きい順に「特異値1〜100位までで近似した場合」を確認。
# 特異値1位〜20位までの間で確認してみる
approximation = []
ks = [1, 5, 10, 20]
for k in ks:
approximation.append(np.dot(np.dot(U, sigma[:, :k]), Vt[:k, :]))
# オリジナル
plt.imshow(grayscale, cmap="gray")
plt.title("original")
# 近似
fig, axes = plt.subplots(nrows=1, ncols=4, figsize=(15,5))
for ax, app, k in zip(axes, approximation, ks):
ax.imshow(app, cmap="gray")
ax.set_title("k = {}".format(k))
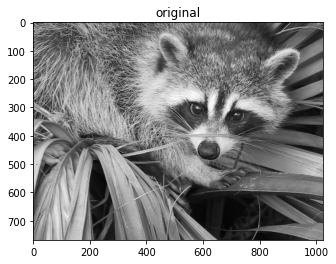
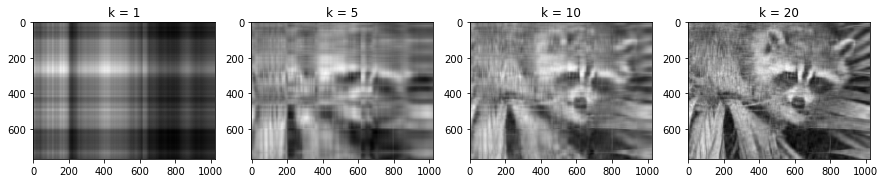